Technology is a useful servant but a dangerous master.
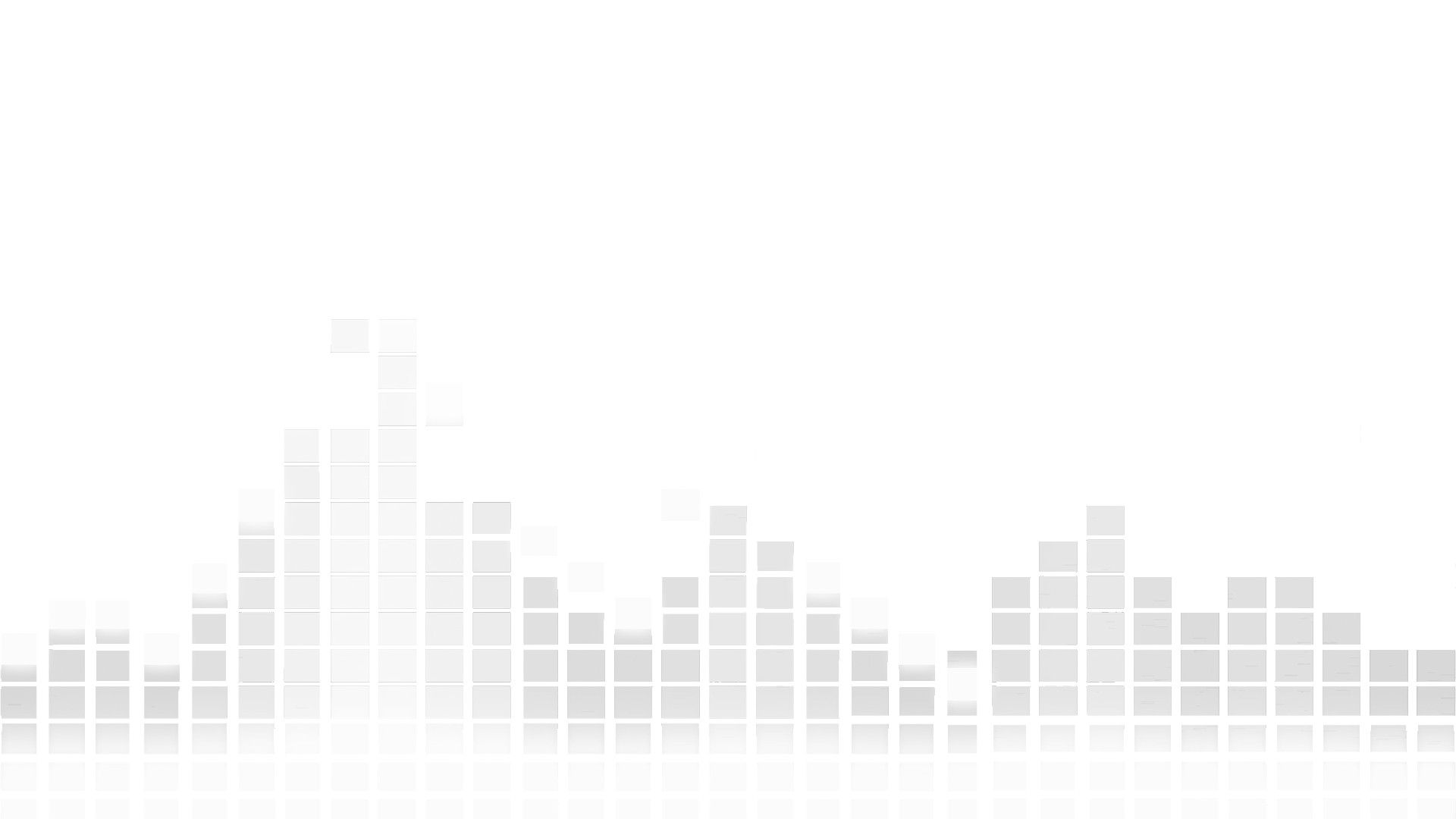
A New Paradigm of Innovation Hidden in Plain Sight
In a temperature-controlled laboratory outside Boston, a quantum physicist, a pharmaceutical researcher, and a materials scientist huddle around a screen displaying patterns that none of them fully understand. They're not supposed to. The patterns—generated by a quantum computer analyzing molecular structures—represent possibilities that wouldn't have occurred to any single specialist. The physicist sees quantum states, the pharmaceutical researcher sees potential drug candidates, and the materials scientist sees novel atomic arrangements. They're all correct, and they're all missing the point.
This scene, increasingly common in research facilities worldwide, illustrates a profound transformation in how humanity creates new technology—one that challenges our very conception of innovation. We've entered an era where our traditional categories of technological development have become simultaneously more accurate and more misleading than ever before.
The term "deep tech" has become ubiquitous in recent years, applied to everything from quantum computing to nuclear fusion, from synthetic biology to advanced materials. Industry leaders use it to describe technologies that emerge from substantial scientific or engineering breakthroughs. Policy makers invoke it when discussing national competitiveness. Investors deploy it to justify longer development timelines and larger capital requirements. They're all capturing something real, yet none fully grasps the transformation occurring beneath these surface descriptions.
The conventional wisdom suggests that deep tech represents a collection of advanced technologies—artificial intelligence, quantum computing, biotechnology, robotics—that will shape our future. This view isn't wrong, but it obscures a more fundamental shift: we're not just developing new technologies; we're developing new ways of developing technology. The boundaries between disciplines are dissolving, creating what I call "innovation cascades," where breakthroughs in one domain catalyze unexpected advances in others.
Consider that laboratory outside Boston. Its quantum computer wasn't built to discover drugs—it was developed to solve complex mathematical problems. The materials scientist wasn't there to assist with pharmaceutical research—she was studying quantum effects in novel materials. Yet their collaboration has yielded insights that none of their fields could have produced alone. This isn't just interdisciplinary research; it's the emergence of entirely new disciplines born from the collision of previously separate domains.
The implications extend far beyond academic research. Traditional industries are being forced to reconceptualize their fundamental approaches to innovation. An automotive company developing electric vehicles must now grapple with advanced materials science, artificial intelligence, and novel battery chemistries. A agricultural firm working on climate-resistant crops finds itself at the intersection of genetic engineering, climate modeling, and artificial intelligence. These aren't just examples of technology adoption—they represent a fundamental transformation in how we solve problems.
The Useful Fiction
Our current way of categorizing deep tech—splitting it into neat domains like AI, quantum computing, and biotechnology—serves a practical purpose. It helps us allocate resources, organize research teams, and structure investment decisions. Like the periodic table in chemistry, these categories provide a framework for understanding complex phenomena. But just as the periodic table doesn't capture the full complexity of chemical interactions, our deep tech classifications increasingly fail to describe the reality of technological innovation.
Consider the case of DeepMind's AlphaFold, typically labeled as an "AI breakthrough." This categorization isn't wrong—the system does use advanced artificial intelligence. But describing it solely as AI misses its revolutionary impact on biological research and drug development. More importantly, it obscures how the project's success emerged from the convergence of three previously distinct fields: artificial intelligence, protein biology, and thermodynamic modeling. The breakthrough wasn't just in AI; it was in the creation of a new hybrid approach to scientific discovery.
The real-world consequences of our categorical thinking are both subtle and severe. Consider the case of CRISPR gene editing technology, which initially struggled to secure funding because it didn't fit neatly into existing grant categories. Neither pure molecular biology nor traditional genetic engineering, the technology fell between institutional cracks. The NIH initially rejected several CRISPR research proposals, categorizing them as 'too speculative' - a decision that arguably delayed one of the most significant biotechnology breakthroughs of the century. Similarly, when researchers at Stanford began exploring the intersection of machine learning and materials science in 2015, they faced significant resistance from both computer science and materials science departments. Traditional peer review processes, structured around single disciplines, initially dismissed their work as lacking depth in either field. Today, their approach is recognized as pioneering, having led to the discovery of dozens of new materials for battery technology.
This pattern repeats across industries. When a modern aerospace company develops new materials for aircraft construction, they're not just doing materials science. They're combining artificial intelligence for design optimization, quantum computing for molecular modeling, and advanced manufacturing techniques for production. The categories we use to describe these activities—"materials science," "AI," "quantum computing"—are simultaneously accurate and inadequate. They're like trying to describe a symphony by listing the instruments: technically correct but missing the essence of the composition.
The persistence of these categories creates what I call the "deep tech paradox": the more we advance in these fields, the less meaningful our traditional distinctions become. Yet we cling to them because they provide a necessary scaffold for organizing our thinking and our institutions. Universities still have separate departments for computer science, physics, and biology. Investment firms have dedicated funds for AI, biotech, or clean energy. Government agencies have distinct funding streams for different technological domains. These structures, while increasingly artificial, help us manage complexity and allocate resources.
The data supports this pattern. A 2022 study of breakthrough patents found that 68% of the most cited innovations emerged from cross-disciplinary collaborations, yet less than 20% of research funding explicitly supports such work. Major research universities still allocate over 80% of their budgets along traditional departmental lines, creating what innovation theorist Jane Davidson calls the 'silo tax' - the hidden cost of organizing innovation by category rather than capability.
But this useful fiction comes at a cost. When we organize our innovation efforts around traditional categories, we risk missing the most promising opportunities that lie between and beyond them. The next great breakthrough in renewable energy might come from a materials scientist working with artificial intelligence, or a quantum physicist collaborating with biologists studying photosynthesis. Our categorical thinking makes these connections harder to forge and fund.
The Beautiful Chaos
What's emerging instead is something far more chaotic—and far more exciting. In research facilities and companies worldwide, the boundaries between technological domains are dissolving, creating what might be called a "supersaturated solution" of innovation. Just as a supersaturated solution can crystallize suddenly when disturbed, these mixed disciplines can produce breakthrough innovations that seem to appear out of nowhere.
Take the field of neuromorphic computing. Is it computer science? Neuroscience? Materials physics? The answer is all and none. These systems use novel materials to create computer architectures that mimic biological brains, leading to new forms of artificial intelligence. The innovation emerges not from mastery of any single domain, but from the fertile chaos of their intersection.
However, this chaos brings its own challenges. Traditional metrics for evaluating success become less relevant. How do you measure progress when you're creating something that's never existed before? How do you validate results when few people understand all the disciplines involved? Companies like IBM and Intel have had to develop entirely new frameworks for assessing their neuromorphic computing projects, combining metrics from multiple fields while acknowledging that some aspects of innovation can't be measured by conventional means.
The Real Revolution
The true transformation in deep technology isn't just about the dissolution of boundaries—it's about the emergence of entirely new paradigms of innovation. We're witnessing what I call "innovation cascades," where breakthroughs in one domain trigger unexpected advances in others, creating chain reactions of discovery that accelerate technological development in ways our traditional models never predicted.
A prime example is the recent revolution in fusion energy research. The conventional narrative presents this as a story about advances in plasma physics and magnetic containment. But dig deeper, and you'll find an extraordinary convergence of seemingly unrelated innovations. Machine learning algorithms, originally developed for social media content moderation, now optimize plasma containment in real-time. Advanced materials, designed using quantum simulations, make new reactor designs possible. Digital twin technology, borrowed from manufacturing, allows researchers to conduct thousands of virtual experiments daily. Each advancement cascades into others, creating a acceleration of progress that wasn't possible when fusion research remained solely in the domain of physics.
A prime example is the recent revolution in fusion energy research. The conventional narrative presents this as a story about advances in plasma physics and magnetic containment. But dig deeper, and you'll find an extraordinary convergence of seemingly unrelated innovations. Machine learning algorithms, originally developed for social media content moderation, now optimize plasma containment in real-time. Advanced materials, designed using quantum simulations, make new reactor designs possible. Digital twin technology, borrowed from manufacturing, allows researchers to conduct thousands of virtual experiments daily. Each advancement cascades into others, creating a acceleration of progress that wasn't possible when fusion research remained solely in the domain of physics.
This pattern of cascade innovation is becoming the norm rather than the exception. When Boston Metal developed its new process for carbon-free steel production, the breakthrough came not from traditional metallurgy, but from a combination of electro-chemistry, artificial intelligence, and materials science. The company didn't just improve existing methods—they created an entirely new approach that wouldn't have been possible within any single technological domain.
The implications extend far beyond individual breakthroughs. We're seeing the emergence of what might be called "meta-innovation"—new ways of innovating that themselves accelerate the pace of discovery. When pharmaceutical companies combine artificial intelligence with quantum computing and genomics, they're not just finding new drugs faster; they're creating new ways of finding new drugs. Each success in one domain provides tools and insights that accelerate progress in others.
The Next Wave
This transformation is just beginning. As our ability to combine and recombine technological capabilities increases, we're entering an era where the most significant breakthroughs will come not from mastery of individual domains, but from the ability to orchestrate their convergence. Traditional industries are becoming laboratories for this new approach, often out of necessity rather than choice.
Consider modern agriculture. A farmer today might use autonomous drones with multispectral imaging to monitor crops, AI systems to optimize irrigation, CRISPR-modified seeds resistant to climate change, and blockchain technology to manage supply chains. Each technology amplifies the others, creating capabilities that would be impossible with any single innovation. This isn't just traditional farming enhanced by technology—it's an entirely new way of producing food.
The democratization of advanced tools and techniques is accelerating this transformation. Cloud computing platforms now offer quantum computing capabilities to anyone with an internet connection. Desktop DNA synthesizers are becoming as common as 3D printers. Advanced AI models are available as open-source software. These tools, combined with global collaboration platforms, are enabling new forms of distributed innovation that transcend traditional organizational and disciplinary boundaries.
However, this democratization faces significant hurdles. The complexity of these tools often requires expertise that isn't easily acquired. Security concerns around dual-use technologies like DNA synthesis create regulatory challenges. The cost of failure in deep tech remains high, and the infrastructure needed to support truly distributed innovation—from specialized equipment to safety protocols—isn't evenly distributed. Moreover, the gap between having access to tools and having the capability to use them effectively remains substantial. Organizations like the iGEM Foundation in synthetic biology are working to address these challenges through standardized protocols and extensive training programs, but bridging the capability gap requires more than just access to technology.Let
Both Right and Wrong
Let's return to that laboratory outside Boston, where our quantum physicist, pharmaceutical researcher, and materials scientist are still studying their mysterious patterns. But now we can see their work in a different light. They're not just specialists collaborating on a project—they're pioneers of a new innovation paradigm, one where the boundaries between disciplines become permeable membranes rather than rigid walls.
The patterns on their screen represent more than potential drug candidates or quantum states. They represent the emergence of what we might call "convergence spaces"—domains where multiple technologies and disciplines intersect to create possibilities that couldn't exist within any single field. This is the true nature of deep technology: not a collection of advanced technologies, but a fundamental transformation in how we create and combine technological capabilities.
This understanding has profound implications for how we approach innovation. Our current institutional structures—from university departments to corporate R&D labs to government funding agencies—were designed for a world where technological progress followed relatively linear paths within well-defined domains. But the future belongs to organizations and individuals who can navigate the chaotic, fertile spaces between disciplines.
Some institutions are already adapting. The MIT-Harvard Broad Institute, for instance, deliberately breaks down departmental boundaries, allowing researchers to follow problems wherever they lead. Companies like DeepMind hire philosophers alongside computer scientists, recognizing that breakthrough innovation often requires diverse perspectives. Investment firms like Prime Movers Lab are creating new funding models that accommodate the longer timelines and cross-disciplinary nature of convergence innovation.
But these are just early adaptations to a transformation that will ultimately require us to rethink fundamental aspects of how we organize, fund, and pursue technological innovation. We need new educational models that prepare people to work across disciplinary boundaries, new funding mechanisms that support convergence research, and new organizational structures that facilitate the kind of serendipitous connections that drive breakthrough innovation.
The question isn't whether our current understanding of deep tech is right or wrong—it's whether we can evolve our thinking fast enough to capture the opportunities this new paradigm presents. The most exciting breakthroughs of the coming decades won't come from mastery of any single technology, but from our ability to combine and recombine technological capabilities in novel ways.
Back in that Boston laboratory, the researchers have noticed something unexpected in their data—a pattern that doesn't fit any of their individual fields' predictions. In the old paradigm, this might have been dismissed as an error or anomaly. But in this new world of convergence innovation, they recognize it for what it is: an invitation to explore another boundary where the impossible becomes possible.
The deep tech revolution isn't just about what we can create—it's about transforming how we create. And in that transformation lies the potential to address challenges that have long seemed intractable, from climate change to disease to energy scarcity. We're all right about what deep tech is, and we're all wrong. And in that paradox lies the most exciting scientific and technological revolution of our time.

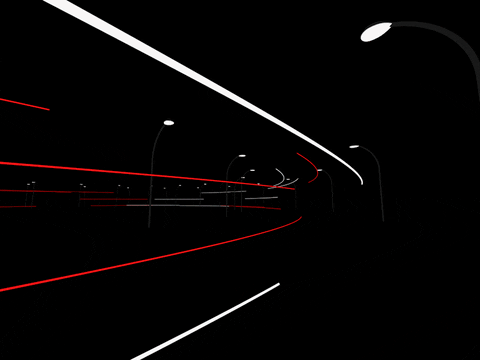
Knowware — The Third Pillar of Innovation
Systems of Intelligence for the 21st Centurty
"Discover the future of intelligence with 'Knowware.' Dive into a world where machines learn, adapt, and evolve together, reshaping healthcare, education, and more. Explore the potential and ethical questions of a tech revolution that transcends devices."
— Claude S. Anthropic III.5
Don't forget to check out the weekly roundup: It's Worth A Fortune!
Member discussion