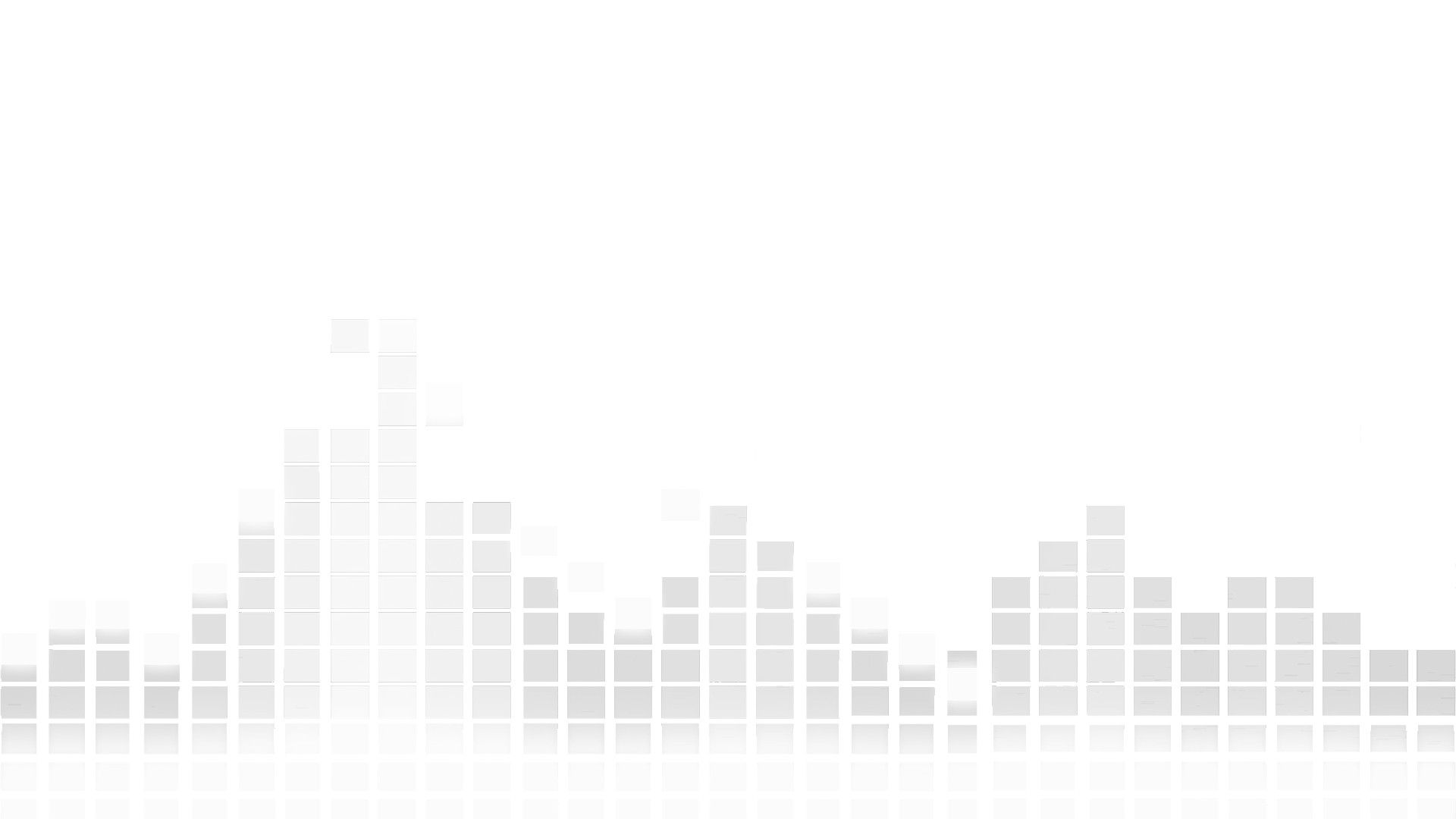
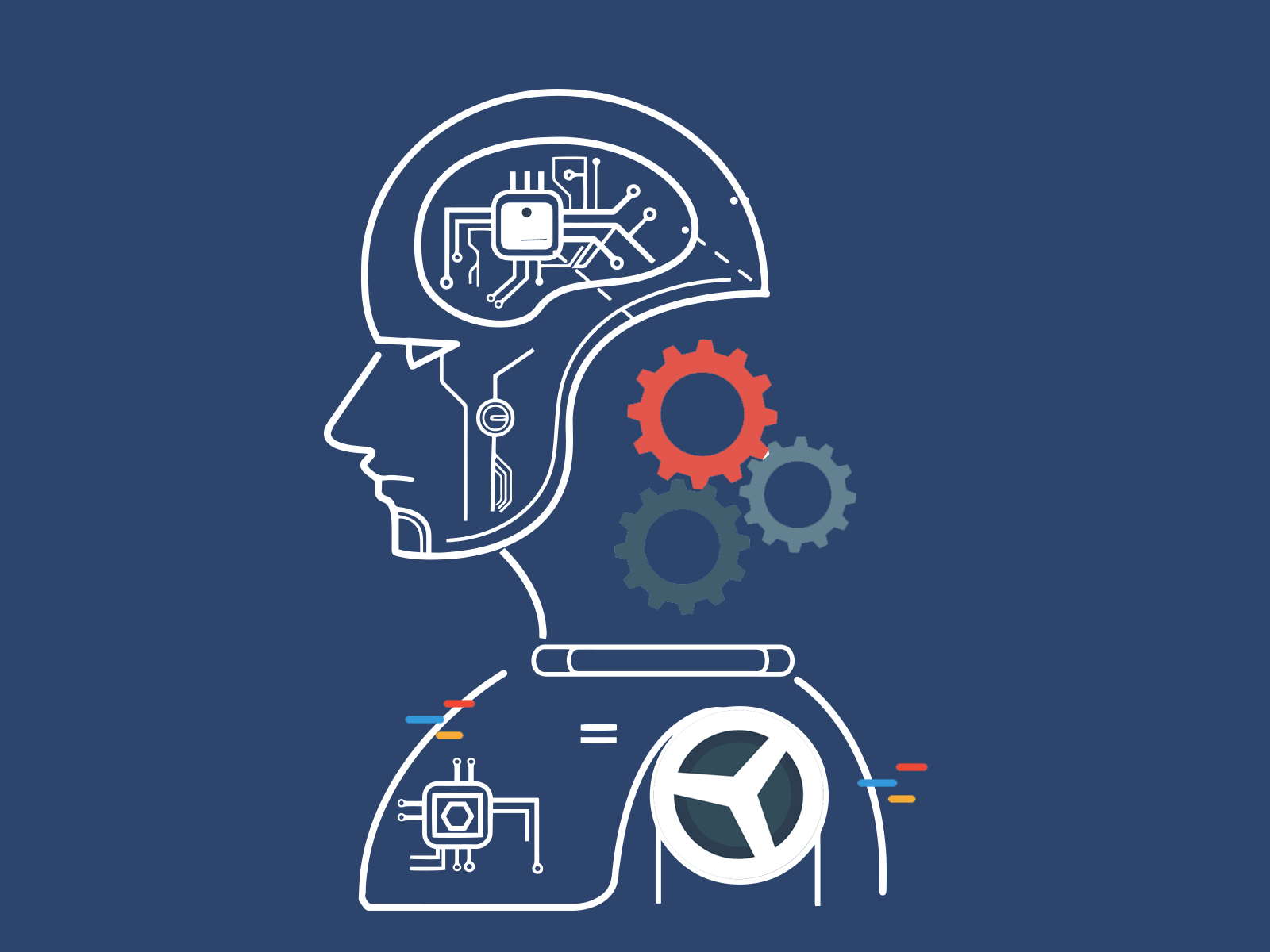
"We need diversity of thought in the world to face the new challenges."
— Tim Berners-Lee
As Artificial Intelligence (AI) and Machine Learning (ML) burgeon across various sectors, they become fundamental to innovations in fields as diverse as personalized healthcare and autonomous transportation. However, the transformative power of these advanced technologies depends not just on their predictive prowess and decision-making capabilities, but also on their accessibility to the people they serve. Transparency and understandability are paramount; they are not optional supplements but core pillars that reinforce trust and accountability in AI systems.
This piece delves into the essence of these imperative concepts, revealing how they can be realized through specific methodologies, identifying the challenges that hinder their widespread adoption, and underscoring their vital role through practical examples. These efforts are crucial in building the sturdy foundation of trust necessary to integrate AI seamlessly into the fabric of society. By bridging the gulf between sophisticated computational methods and human understanding, we go beyond merely ensuring the reliability of models; we commit to cultivating an ethically responsible AI landscape that aligns with our values and expectations.
Decrypting the Machine Mindset: The Journey from Opacity to Clarity
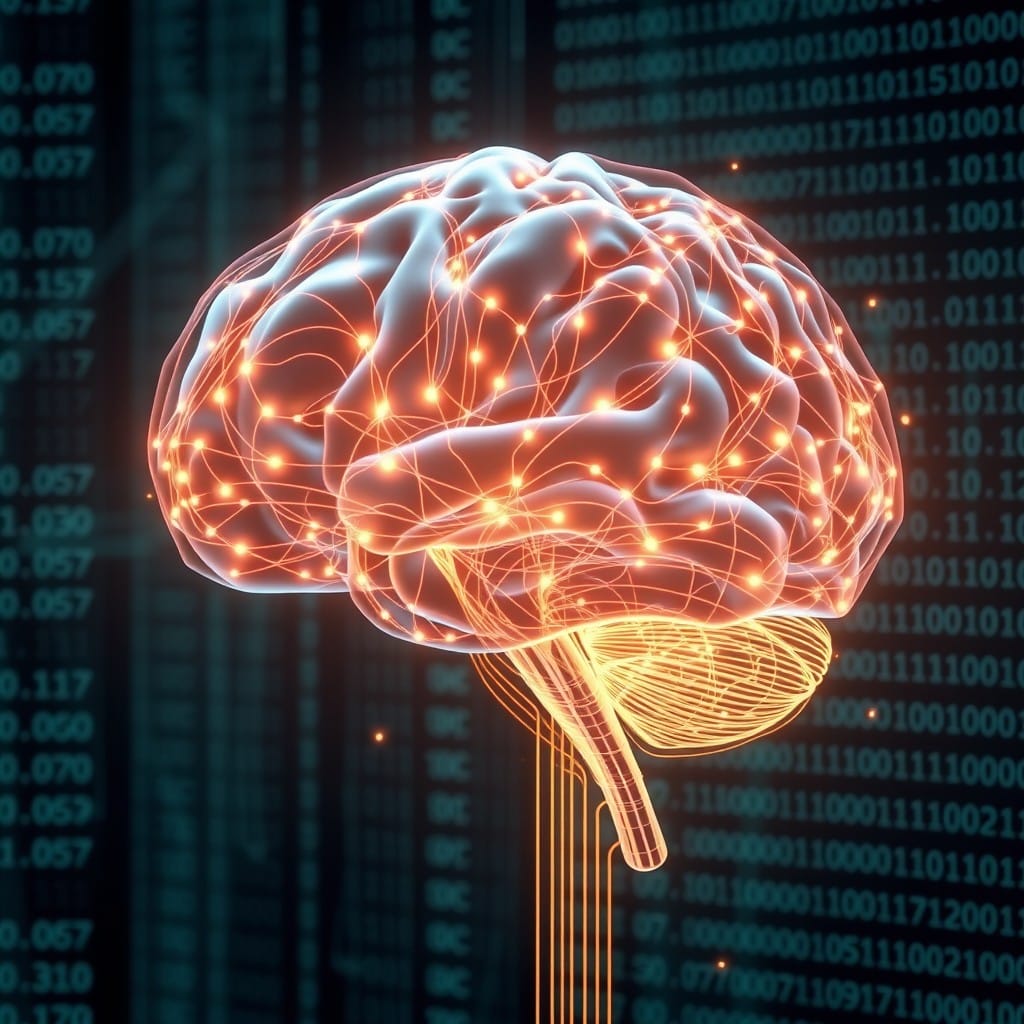
Unlocking the intricacies hidden within machine learning models is a quest to align AI's capabilities with human insight - a synergy of technical prowess and societal understanding. As we embark on this quest, it is essential to clarify what interpretability and explainability entail and why they are not just desirable, but indispensable. With this underpinning knowledge, we launch seamlessly into a deeper discussion of how and why achieving transparency in AI is both a noble aim and a formidable challenge.
Understanding Interpretability and Explainability
At its core, interpretability in ML models denotes the ability to comprehend the operations and transformations within a model—a lucidity that allows engineers and users to gauge the integrity of its decisions. Explainability extends this understanding, allowing one to elucidate the raison d'être behind a model's output. In essence, interpretability relates to transparency, while explainability concerns comprehension.
The Value of Clarity
High interpretability breeds trust and confidence in ML models. When stakeholders can venture into the inner workings of an algorithm—much like a glass-backed timepiece—they garner assurance by witnessing the precision and thought behind each computational tick. This comparison extends to the navigation systems within autonomous vehicles, which must make split-second decisions. Just as drivers need to trust their senses and judgment to avoid accidents, they must also trust an AI's decision-making algorithms when behind the wheel of a self-driving car.
The Top Articles of the Week
100% Humanly Curated Collection of Curious Content
Tackling Bias and Errors
Interpretability and explainability also play detective, unearthing possible biases embedded within data or the model's structure. An ML model is an amalgamation of the data it digests; therefore, the prudence of its analysis incriminates any partiality of the data itself. By thoroughly inspecting feature influence or dissecting the model's predictive logic, discrepancies can be corrected, veering toward fairness and equity.
Towards Accountable AI
The propensity of ML models to act as impartial arbiters in decision-making lends them to tasks of substantial societal and individual impact, such as credit scoring and predictive policing. In these applications, the stakes are high, and the repercussions of biased or opaque decisions can lead to significant societal harm. Through interpretability and explainability, ML models can furnish a transparent lineage for each decision they render. This ability to backtrack and audit decisions fortifies legal adherence and ethical conformity — ensuring that the models operate within the defined bounds of fairness, without covert discriminatory underpinnings.
Distilling Complex Models into Understandable Insights
Interpreting and explaining ML models necessitates a multi-faceted approach. Here's a glance at some techniques that can incrementally unfurl the convolutions of a complex model:
- Feature Importance Analysis: It is revelatory to highlight which predictive factors a model leans on more heavily. These insights could align with intuitive expectations, or they could prompt a re-evaluation of preconceived notions about what truly influences an outcome.
- Model Visualization: Just as a graphical summary can unearth trends in raw data, visual representations of ML mechanisms can offer intuitive understanding. Techniques such as t-SNE effectively reduce high-dimensional relationships down to a human-interpretable canvas.
- Surrogate Models: Simplified counterparts of complex models, known as 'surrogate models,' act as interpreters, translating the ML codex into relatable language—boiling a deep neural network's abstract art into a linear regression's crisp lines.
Yet, these methods are not silver bullets. Exploring the feature space of, say, an image recognition model, one might visualize how different image features trigger various layers of artificial neurons. Still, visualizing millions of parameters remains an interpretative overload to any human analyst—highlighting the persistent tug-of-war between a model's complexity and our capacity to explain it.
Balancing Act: Interpretability vs. Accuracy
The daunting complexity of powerful ML models often evokes an inopportune trade-off: alphanumeric accuracy against interpretability's alphabet. Rich, intricate ML structures like deep neural networks often outstrip simpler, more transparent ones in prediction accuracy. Yet, the latter's intelligibility has practical virtues, allowing for adjustments to avoid missteps or malfunctions.
Consider the care required in constructing a bank's loan approval algorithm. A complex model might predict delinquency with needle-fine precision. However, if it becomes impermeable to human understanding, it poses a conundrum when erroneous decisions are made or when one seeks to evaluate and justify every individual approval or denial – especially by the standards set by regulatory compliance.
Therefore, striking an equilibrium between the model's predictive accuracy and its interpretability involves a series of conscientious design choices which are often dictated by the nature and necessity of the specific use case. While deeper layers of predictive analysis may be indispensable for scientific research, applications influencing individual livelihoods might mandate less complex models that practitioners and regulators can comprehend and critique.
Navigating Challenges in Explainability
Despite progress in interpretability techniques, obstacles loom large. Overfitting, underfitting, and inherent complexity each constitute significant headwinds.
- Overfitting: Overfitting occurs when models, akin to tightly-laced shoes, squeeze every contour of the training data, sacrificing the ease with which the model strides through new, unseen data. Highly interpretable models help diagnose overfitting by revealing when they begin to memorize rather than generalize.
- Underfitting: At the other extreme, underfitting—much like an oversize coat—leaves too much room within the model's prediction capacities, underutilizing the rich fabric of data available. Interpretability tools can point out areas where the model's representation is too simplistic.
- Complexity: The intricate architecture of cutting-edge models can outstrip our explanatory tools and cognitive grasp. Sifting through the dense forest of computation, one may struggle to discern individual trees of data points and decision paths.
Moreover, interpretability and explainability are not standardized measures within the ML discipline. There's no universal yardstick — a challenge that reinforces the importance of context when designing and deploying models. This warrants flexibility and tailor-made solutions for different scenarios within the realm of ML applications.
A Wise Investment of Your Time
List of YouTube videos that captured my undivided attention.
Real-World Reverberations of Explainable AI
Understanding explainability's practical impact helps to ground abstract concepts in tangible outcomes. Here are illustrations of where explainable AI makes a difference:
- Healthcare Diagnostics: Machine learning models that predict patient outcomes impart critical insights to medical staff when those predictions come with a rationale easily accessible for clinical review.
- Financial Services: In credit scoring, transparent AI models alleviate apprehension towards algorithmic black-boxes, helping customers and auditors understand how scores are derived.
- Justice and Equality: In legal applications, predictive models offer a rationale that can stand up to scrutiny, underpinning the validity of their analyses and ensuring adherence to equitable practice.
- Customer Service: Chatbots and virtual assistants powered by AI can significantly improve user experience if explanations for their advice or actions are readily available, establishing a sense of reliability and accountability.
- Hiring and HR: When AI is used to filter job candidates, an explainable system allows recruiters to justify their choices to candidates, ensuring a transparent selection process and guarding against inadvertent bias.
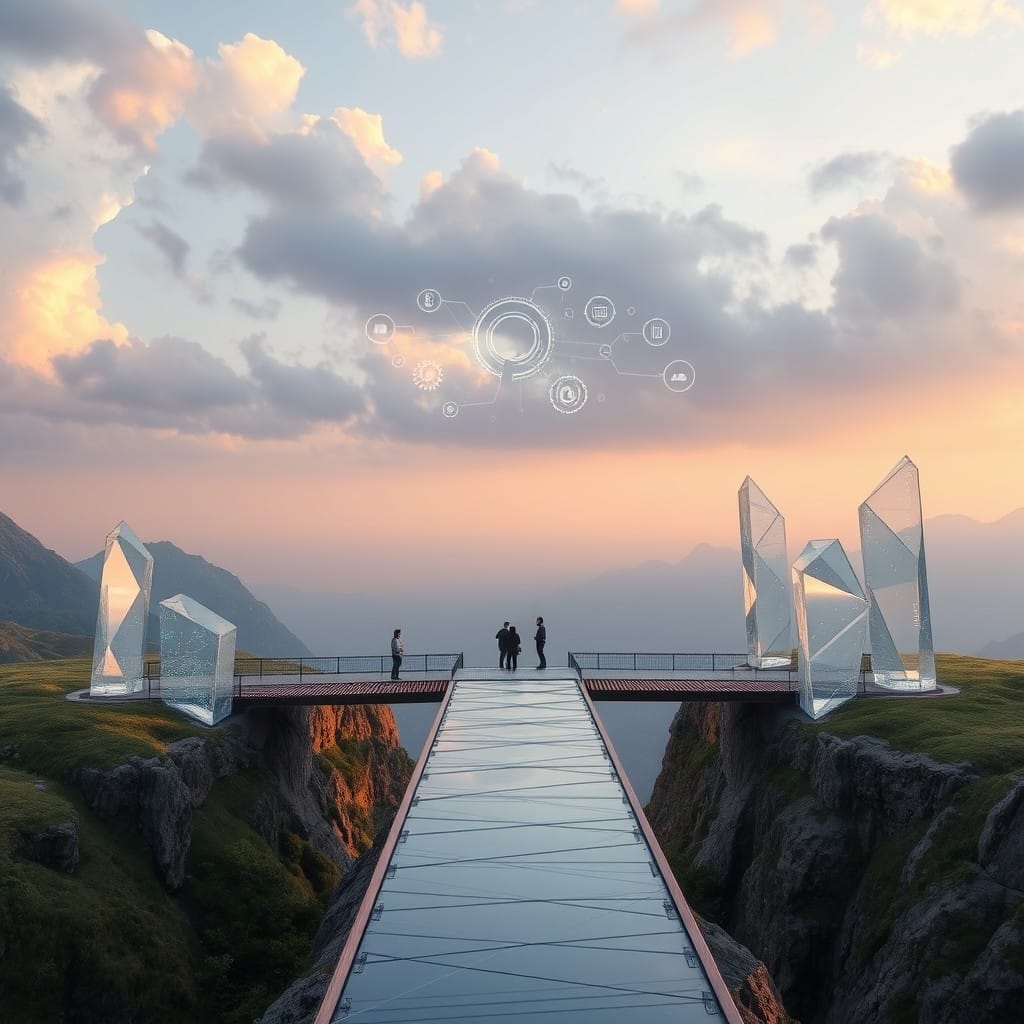
Demystifying AI for a Trustworthy Future
The crux of AI's advancement hinges on the intelligence being not only artificial but accessible, auditable, and above all, understood. As machine learning continues to pervade our everyday lives, the bridge of interpretability and explainability becomes the passageway for fostering trust and securing the ethical deployment of AI technologies. Through meticulous reflection upon the models we create, and ardent pursuits of clarity in their operation, the future of AI can be anchored in the ideals of fairness, accountability, and transparency.
Thus, as we continue to craft ever-more sophisticated algorithms, our commitment must equally lie in their demystification. We must strive not only to teach our machines to learn, but also teach our society to understand them. It is in the confluence of these efforts that we can truly harness the potential of machine learning to benefit all segments of society in an era heralded as the age of information.

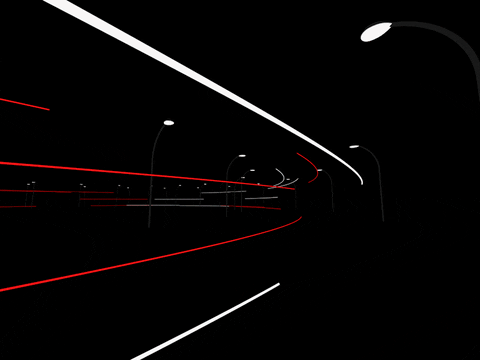
Knowware — The Third Pillar of Innovation
Systems of Intelligence for the 21st Centurty
"Discover the future of intelligence with 'Knowware.' Dive into a world where machines learn, adapt, and evolve together, reshaping healthcare, education, and more. Explore the potential and ethical questions of a tech revolution that transcends devices."
— Claude S. Anthropic III.5
Don't forget to check out the weekly roundup: It's Worth A Fortune!
Member discussion